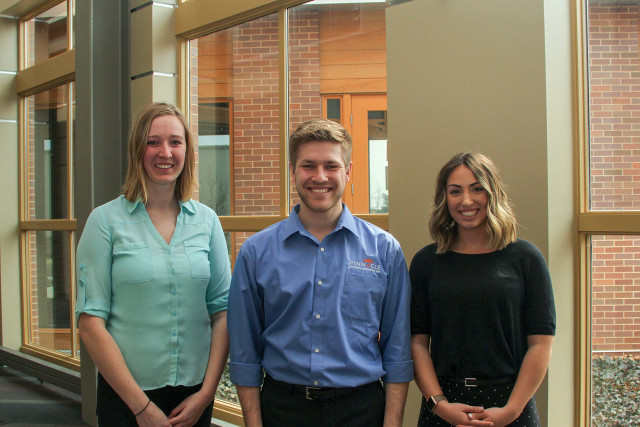
Personalization of Insurance
Co-authored by Brittany Henrich and Joey Sveda.
New technologies that gather personal information are changing the manner in which prices are set by insurance companies. Telematics, wearables and smart home features are but three examples of technologies personalizing the business of insurance.
The proliferation of outlets to secure insurance coverage (traditional agents, direct mail, internet portal, smart phone app) have led to advancements in technology and increasing competition, both of which allow consumers to quickly seek out personalized insurance options.
During the recent Pinnacle University event, we discussed the topic of personalized insurance and its place in the insurance market. The topic of personalized insurance includes the use of more personal data and predictors to price insurance for specific customers as well as the ability to find insurance coverage for virtually anything you need at any limits you may desire. One interesting example of personalized insurance is a celebrity insuring their body parts, such as David Beckham insuring his legs for £100 million. Is it possible to get more personalized than that?
Our presentation focused on insurers’ ability to use personal data and predictors in pricing insurance. Historically, insurance rates have been based on traditional predictors such as age, gender, marital status, geographical location and credit score. Thanks to advancements in technology, insurers are collecting more data up front to be used as predictors of future claim potential.
Telematics data is helpful in assessing driver patterns. Wearables (such as Fitbits) can track an insured’s mobility and overall health patterns to assess the frequency and/or severity of future health or workers’ compensation claim potential.
Recent developments in pricing commercial auto (e.g., trucking) insurance may allow insureds to buy coverage based on each delivery instead of purchasing an annual policy. This per-delivery pricing structure can calculate the real-time risk based on the specific driver, type of truck, weather conditions and roads travelled in such a way to personalize the cost of insurance based on detailed predictors.
But where and when does it end? Is it possible that an individual’s DNA from Ancestry.com will be used to price health insurance? Might family history factor into the final premiums you must pay? Too much personalization runs the risk of not only reviewing predictors that may not be statistically significant, but also run afoul of consumer protection laws.
As the quantity and availability of data increases, actuaries are using more sophisticated statistical methodologies to gain competitive advantages. The most common of these methodologies is Generalized Linear Modeling (GLM). The GLM process essentially analyzes data from multiple risk variables simultaneously. Traditional univariate actuarial methods that considered variables one at a time are not able to account for the correlation of variables.
GLM is easy to interpret and provides results that are acceptable to most regulators. GLM helps us understand what predictors are significant and which are not. Lastly, GLM provides us with evidence of correlation versus causation. For example, consider the intersection of ice cream, summer weather and heart attacks. Just because there may be a correlation between the sale of ice cream and heart attacks in the summer, ice cream does not cause heart attacks. Rather, GLM would test all pertinent hazards together in order to remove predictors that are not statistically significant.
The Actuarial Standard of Practice #12 on Risk Classification (Section 3.2.1) states,
“Rates within a risk classification system would be considered equitable if differences in rates reflect material differences in expected cost for risk characteristics. In the context of rates, the word fair is often used in place of the word equitable.”
There has been a trend towards state insurance regulators imposing harsher restrictions on the allowable risk classification predictors in the insurance ratemaking process, despite actuarial justification that may be provided as evidence in support of such. The state of California recently decided to restrict the use of gender in automobile insurance rates. There has been discussion in New York and Maryland about the ability to use education and occupation as predictors. Also, the US House of Representatives just held a hearing on the use of credit scores. Hawaii, although not a new trend, restricts an insurer’s use of a person's age, gender, length of driving experience, credit bureau rating, marital status and/or physical handicap within rating plans.
As the insurance market continues to evolve, new opportunities for personalizing insurance will continue to appear and face new challenges as the market strives to reach a balance. State regulators will continue to examine insurers’ risk classification plans to protect consumers.
Brittany Henrich is an Actuarial Analyst 2 with Pinnacle Actuarial Resources, Inc. in the Bloomington, Illinois office. She holds a Bachelor of Science degree in Actuarial Science from the University of Illinois in Urbana-Champaign. Brittany has experience in assignments involving Loss Reserving, Loss Cost Projections and Group Captives. She is actively pursuing membership in the Casualty Actuarial Society (CAS) through the examination process.
Joey Sveda is an Actuarial Analyst with Pinnacle Actuarial Resources, Inc. in the Bloomington, Illinois office. He holds Bachelor of Business Administration degree in Actuarial Science from the University of Wisconsin - Madison. He has experience in assignments involving Loss Reserving, Group Captives and Predictive Analytics. Joey is actively pursuing membership in the Casualty Actuarial Society (CAS) through the examination process.